For almost a decade, development organizations have used digital human behavioral data, or “digital crumbs,” to gauge responses to the increasingly complex development challenges of the 21st century. Large quantities of data generated from social media interactions, financial transactions, or mobile phone usage allow for insights into people’s behaviors, movements, and choices. When analyzing such data, the common practice has been to focus on the aggregate — the collective behavior of individuals and groups — while discarding extreme observations, or outliers. In cases where outliers are analyzed, the focus is typically on negative outliers such as crime hotspots or high deforestation areas.
But what if we looked into positive outliers instead, attempting to understand the underlying factors and practices leading to favorable outcomes?
Positive outliers matter
This is the goal of our Data Powered Positive Deviance initiative. Created by organizations with extensive experience in the data for development space as an open testing and learning network, we explore the use of new digital data sources in the systematic identification and understanding of positive outliers in various domains. We do this by building on a development approach referred to as Positive Deviance.
Based on the observation that in every population there are individuals or communities who, despite facing similar challenges and limitations, achieve better results than their peers, this approach focuses on these outliers (or positive deviants) in order to discover unusual practices and strategies that successfully solve complex problems – particularly where conventional solutions failed. Positive deviants might be farmers with better yields than their neighbors; parents who keep their children well-nourished when most are under-fed; loggers who maintain carbon stocks when others are deforesting; or communities that perform significantly better in containing a pandemic such as Covid-19.
From Positive Deviance to Big Data-Based Positive Deviance
Despite the proven success of the conventional Positive Deviance approach in several countries and sectors, there are challenges limiting its widespread adoption. The approach relies heavily on primary data collection to develop a baseline from which positive deviants are identified — a process that is both time and labor-intensive with costs directly proportional to sample size. As a result, data samples are often relatively small, making positive deviants rare and difficult to statistically identify. Furthermore, the generalization of such practices is challenging, considering the small number of positive deviants. Readily available digital data — satellite imagery, social media data, or data captured by wearable or other IoT devices, for example — could reduce the time, cost, and effort needed for data collection while providing larger sample sizes. Such data sources could also enable positive deviant identification at temporal and spatial scales not previously possible using the conventional approach.
Researchers at the University of Manchester are currently exploring the promise of big data-based positive deviance, and through this initiative we are joining forces in an attempt to unlock its full potential. This endeavor will likely involve the use of various big data sources in order to identify positive deviants, defined as individuals or groups performing unexpectedly well in a specific outcome measure that is digitally recorded, mediated, or observed. For instance, instead of using ground surveys to identify farmers with a much higher crop yield within a village or community, we could utilize vegetation indices derived from earth observation data to identify clusters of high-yield farmers across the country.
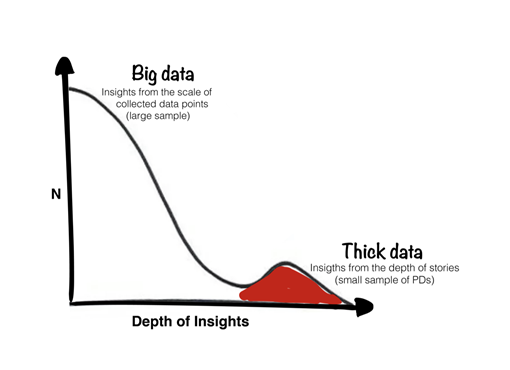
Big data vs. thick data. Adapted from “Why big data needs thick data”
Additionally, we will seek to combine big data with thick data — data that is collected through qualitative and ethnographic methods to uncover individual behaviors and attitudes — to leverage the specific value of both data types. While we can use large samples from big data sources to identify positive deviants, we can view thick data as much more granular, context-rich qualitative information to help us understand the underlying reasons behind deviant practices and behaviors.
Our approach is intentionally designed in a way that does not undermine the importance of ethnographic methods in positive deviance inquiry. Instead, it attempts to integrate traditional and non-traditional data sources for the purposes of identifying, understanding, and scaling uncommon but successful behaviors and practices of positive deviants.
The Initiative
Data Powered Positive Deviance is a global initiative collaboratively created by GIZ Data Lab<Pulse Lab Jakarta, UNDP Accelerator Labs Network, and the University of Manchester Centre for Digital Development. Over the next several months, we will run pilots in various countries to see if and how we might use big data-based positive deviance to tackle development challenges in such sectors as infectious disease control, urban planning, deforestation, and agriculture. We aim to generate a shared understanding, along with a set of tools and techniques, that will allow for the use of a variety of data types and sources in identifying positive deviants across different contextual circumstances.
Along the way, we will share our discoveries, inviting others to jointly explore the emerging field of digital data analysis to strengthen and complement asset-based approaches to finding effective development solutions. The frameworks, tools, and code we plan to develop will be made available for anyone to use and build upon.
Changing Our Approach to Development
What seems to be a very technical concept we consider one of the many ways of shifting from top-down identification and tackling of development challenges to a focus on a community’s inherent assets and capabilities as the ultimate starting point in the search for solutions. It’s an attempt to gradually move away from the imposition of external solutions to the diffusion of local practices and strategies that take into account contextual variables, making them more likely to stick and less vulnerable to social rejection. This goal certainly seems like a tall order but, after observing the initial pilot results, we believe our approach holds immense promise for valuable insights and learning.
Watch this space for updates or get in touch with us by email:
- GIZ Data Lab: Catherine.Vogel@giz.de
- Pulse Lab Jakarta: Dharani.Burra@un.or.id
- UNDP Accelerator Labs Network: Jeremy.Boy@undp.org
- The University of Manchester Centre for Digital Development: Basma.Albanna@manchester.ac.uk